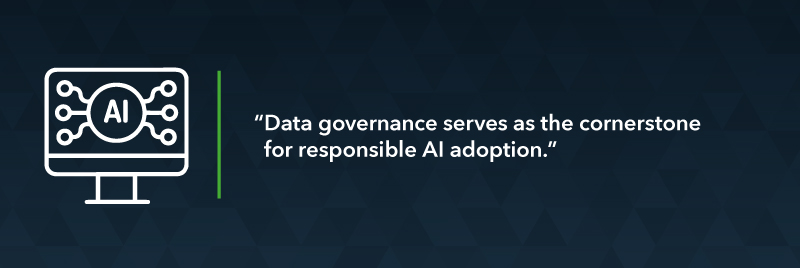
In my role as the Group Head of Data with Ignition Group, I’m often asked what role data governance plays in the adoption of artificial intelligence (AI) technologies. It’s an important question, and one that every business needs to be on top of if they are planning to implement an AI solution.
Before we can answer that question, though, we need to take a closer look at what data governance is and the role of the data team in an enterprise like Ignition Group, which plays a significant role in driving the digital economy in South Africa as we use technology to offer value to our partners and customers.Â
Data governance refers to the management, compliance and usage policies and procedures that organisations use to control, protect and enhance the value of their information. This concept encompasses the practices, guidelines, and standards that ensure it is accurate, accessible, consistent and secure.
The role of a department responsible for data governance is to advocate for a robust framework that guarantees the utmost quality of information availability and unleashes its potential to drive value throughout the business. The mission of the Group Data Team at Ignition, for example, is to empower the Group with trusted metrics and insights, unlocking opportunities, enhancing customer experiences, optimising processes and delivering value to stakeholders. This encompasses cultivating a data-centric culture within the Group, fostering collaboration across departments and championing the ethical and responsible use of customer data touchpoints to create value.
At the core of the Ignition Group data strategy lies a robust data management framework designed to ensure quality, accessibility, value, security and compliance. This framework outlines a comprehensive approach for enhancing data governance and management across its subsidiaries and business units, ensuring alignment with best practices, policies and regulatory standards. The framework defines data as a critical asset essential for executing the Group’s strategy and aims to promote optimal decision-making and foster a data-driven culture within the Group. The following key pillars are:
- Governance
- Establishing clear roles, responsibilities, and processes for managing data assets, including its ownership and stewardship, policies and compliance with regulatory requirements.
- Platform
- Enhancing platforms that integrate multiple data sources, enabling real-time processing, supporting advanced analytics and machine learning.
- Quality
- Implementing procedures and tools for data cleansing, validation and enrichment to maintain high data quality standards across the organisation.
- Security and Privacy
- Enforcing security measures, encryption protocols and access controls to safeguard sensitive data and ensure compliance with data privacy regulations.
- Monetisation
- Unlocking the value of our data assets is a key priority. To this end, we are exploring various monetisation strategies, advanced analytics, machine learning and artificial intelligence, which are critical for most companies to survive in this digital economy.
Now that we’ve all got a handle on what data governance is, and the wide scope and application of it in a technology company such as ours that plays across multiple sectors through our diverse business units, we can begin to look at how it is pivotal in utilising the full potential of AI while minimising associated risks by ensuring the following:
- Quality Data Input
- AI technologies heavily rely on information for training and decision-making. With robust data governance practices in place, we can ensure the accuracy, completeness and relevancy of these inputs used in AI models. This enhances the quality of insights generated and the reliability of AI-driven decisions. To put it simply, the outputs of an AI programme are only as good as the data inputs.
- Ethical AI Use
- Data governance frameworks help establish guidelines for analytics development and deployment. By defining rules for data collection, usage and privacy protection, we can ensure that AI algorithms operate within ethical boundaries, mitigating the risk of bias or discrimination.
- Compliance and Regulation
- In an increasingly regulated environment, adherence to data governance standards ensures compliance with protection laws such as POPIA and specific regulations. By proactively addressing compliance requirements, we safeguard against legal liabilities and reputational damage.
- Risk Management
- Data governance enables us to identify and mitigate risks associated with AI implementation. By accessing information quality, security vulnerabilities and potential algorithmic biases, we can pre-emptively address issues and minimise the likelihood of adverse outcomes.
- Data Security
- Strong data governance measures safeguard against data breaches and unauthorised access, ensuring the security and confidentiality of sensitive information used by AI systems. This fosters trust and safeguards against reputational harm.
- Lifecycle Management
- Effective governance encompasses the entire data lifecycle, from collection and storage to usage and disposal. By implementing clear protocols for data management and retention, we ensure the integrity and longevity of data assets, supporting sustainable AI initiatives.
In essence, data governance serves as the cornerstone for responsible AI adoption, enabling us to leverage AI’s transformative potential while upholding ethical standards, regulatory compliance and risk mitigation strategies. By prioritising it, we can unlock the full value of AI while safeguarding against potential pitfalls.
Naseema Nosarka is Group Head of Data at Ignition Group.
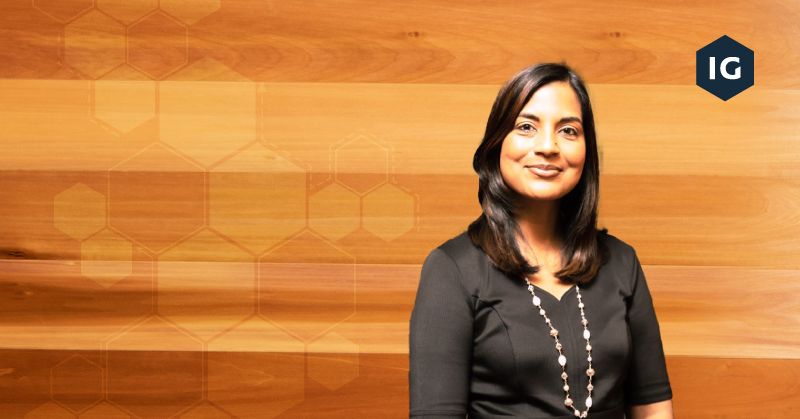